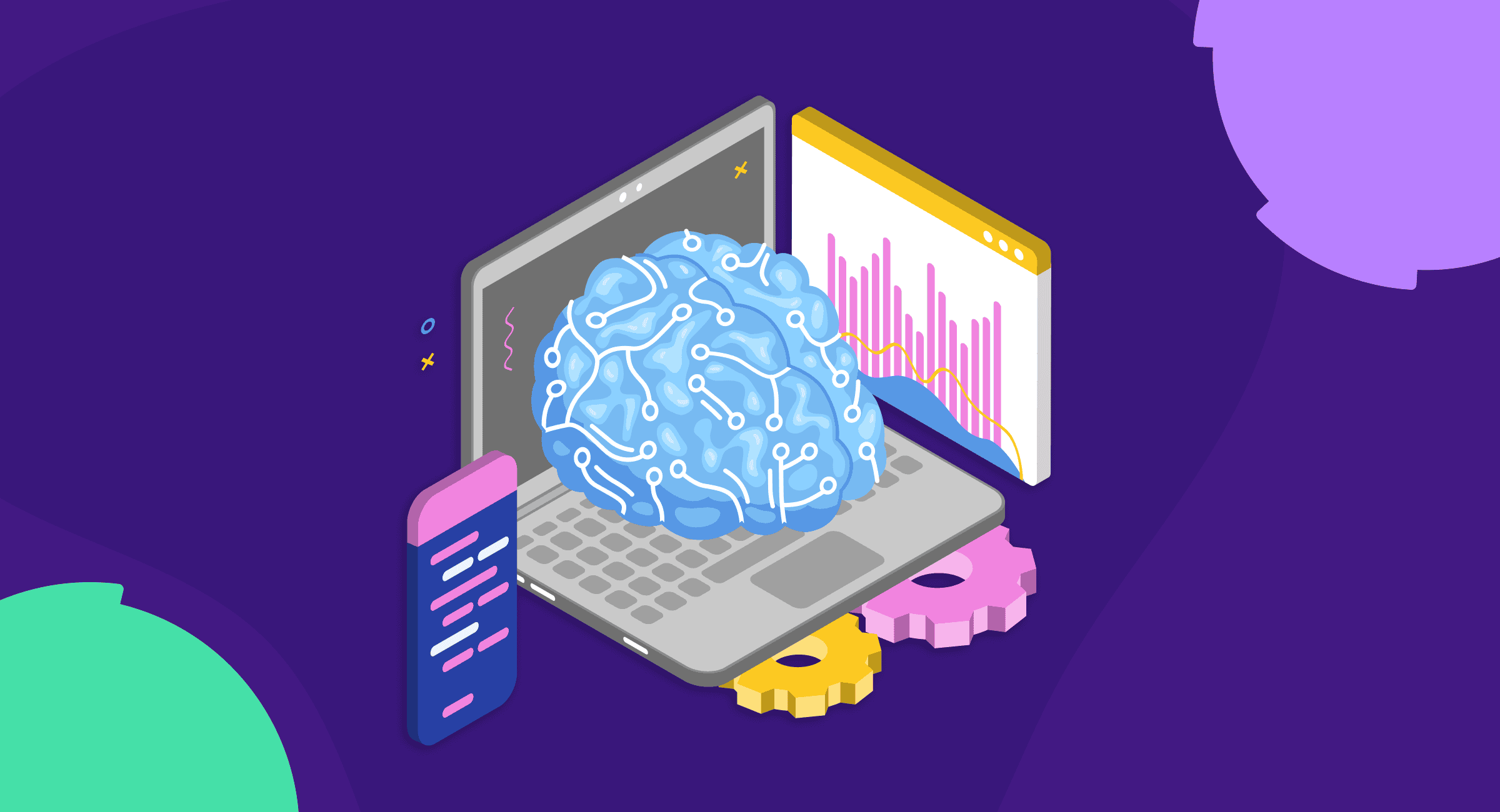
AI is reshaping digital marketing faster than you can say “algorithm update.”
Sophisticated AI models are changing how we approach everything from keyword research to content creation. These aren’t just buzzwords or distant future tech. They’re here now and impacting our daily work.
In this guide, I’ll explain the most relevant AI models for digital marketing and explore their practical applications, benefits, and limitations. My goal is to equip you with the knowledge to confidently ride the new AI marketing waves.
Let’s start with the definition of an AI model and a quick primer on how they work.
What Is an AI Model?
An AI model is a computer program designed to learn from data and make decisions or predictions. Think of it as a digital brain trained to perform specific tasks. These models analyze patterns in vast amounts of information to generate insights, recognize trends, or create content.
AI models work behind the scenes in many aspects of our daily lives. They power the facial recognition that unlocks your smartphone, the voice assistants that schedule your appointments, and the recommendation systems that suggest your next Netflix binge.
They’re the invisible force behind personalized ads, spam filters, and even the autocomplete function in your email and text messages.
Marketers use AI models to:
- Understand and reach audiences
- Analyze consumer behavior
- Understand search intent
- Generate content
- Optimize ad placements
- Personalize user experiences
- Automate SEO tasks
- Predict algorithm changes
But AI isn’t a magic wand. It’s a powerful tool that requires human expertise to use it effectively and ethically.
How AI Models Work
At their core, AI models are pattern recognition machines. They learn by processing large amounts of data and identifying relationships within it.
Here’s a simplified breakdown of how they work:
- Input: The model receives data. This could be text, images, numbers, etc.
- Processing: The model analyzes this data using complex mathematical algorithms. It looks for patterns and relationships.
- Learning: As the model processes more data, it refines its understanding of these patterns. This is called training.
- Output: Once trained, a model can make predictions or decisions when given new, similar data.
Think of an AI model like a child learning to recognize animals. At first, the child might only know dogs. As they see more animals, they learn to distinguish cats, birds, and fish. Eventually, they can identify animals they’ve never seen before based on similarities to ones they know.
AI models work similarly, but on a much larger scale and with more complex data. They can find patterns humans might miss, especially when dealing with large amounts of information.
In digital marketing, these models analyze user behavior data to predict which customers are likely to make a purchase. Or they could process millions of web pages to understand what makes content rank well in search engines.
The “intelligence” in AI comes from its ability to improve over time. As AI models encounter more data, they “learn” and become more accurate.
Now let’s talk about some specialized kinds of AI models.
5 Types of AI Models
The design of many AI models is to tackle specific types of problems. In this section, I’ll highlight the main categories of AI models and show you the marketing challenges they’re best suited to solve.
1. Classification Models
Classification models are the sorting experts of the AI world. These models are designed to categorize data into predefined classes or categories. They analyze input features and decide which category the input belongs to.
In digital marketing, classification models excel at tasks like detecting email spam, and separating legitimate messages from unwanted noise.
Sentiment analysis is another key use, where models categorize customer feedback or social media mentions as positive, negative, or neutral. This helps brands quickly gauge public opinion and respond to issues.
Classification models can also predict customer behaviors, like whether a user is likely to click on an ad or make a purchase. These models can help you make strategic decisions so your marketing campaigns are more targeted.
Classification models are powerful, but require careful training with balanced, representative data to avoid biases in their categorization and predictions.
2. Regression Models
Regression models are the workhorses of predictive analytics. Designed to forecast continuous numerical values, these models establish relationships between variables to predict outcomes based on input data.
In practice, regression models analyze historical data to identify trends and patterns. For instance, they might examine factors like past sales, economic indicators, and seasonal trends to predict future revenue. In digital marketing, you can use them for tasks like SEO forecasting, estimating customer lifetime value, or predicting the number of conversions to expect from an ad campaign.
Like all other AI models, regression specialists have weaknesses. They typically assume linear relationships between variables, which isn’t always the case in marketing. That’s why we often combine them with other techniques for more robust predictions.
3. Clustering Models
Clustering models are the data organizers of the AI world. They find patterns and group similar data points without needing predefined categories.
These models work by analyzing multiple features of each data point and identifying natural groupings. For example, in customer segmentation, a clustering model might consider factors like purchase history, browsing behavior, and demographic information to group customers with similar profiles.
This approach is valuable in digital marketing for tasks like audience segmentation, where you can tailor content and strategies to specific customer groups. However, it’s important to note that while clustering models can reveal hidden patterns, interpreting these clusters often requires human expertise to derive actionable insights.
4. Neural Networks and Deep Learning
Neural networks and deep learning models represent the cutting edge of AI. The technology mimics the human brain’s structure to process complex data. These models consist of interconnected layers of artificial neurons (yes, just like our brains), and each neuron can learn and adapt based on the input it receives.
Deep learning refers to neural networks with many layers. These types of networks can tackle highly sophisticated tasks.
In digital marketing, these models have revolutionized how we handle rich media and unstructured data. They power image recognition systems that can analyze visual content in ads or user-generated images and provide valuable insights about brand perception and consumer preferences.
These models also power speech recognition, which enables voice search optimization, and chatbots that understand natural language. Perhaps most impressively, deep learning models can generate human-like text, which has opened new frontiers in content creation and personalization.
5. Natural Language Processing (NLP) Models
Natural Language Processing (NLP) models are AI’s linguists. They’re designed to understand, interpret, and generate human language. These models bridge the gap between human communication and computer understanding, and enable machines to read, decipher context, and even produce text that mimics human writing.
At their core, NLP models analyze the structure and meaning of language. They can identify sentiment in customer reviews, extract key information from documents, or generate human-like responses in chatbots.
Large Language Models (LLMs), a powerful subset of NLP models, have taken these capabilities to new heights. They can produce coherent articles, answer complex questions, and even engage in creative writing tasks.
In digital marketing, NLP models power chatbots that provide 24/7 customer service, analyze social media sentiment to gauge brand perception, and assist in content creation by generating ideas or even drafting entire articles.
There is overlap between deep learning and language processing technologies, because many of these advanced NLP models, especially LLMs, are built on neural network architectures.
Popular AI Models: 5 Examples
The AI landscape is enormous, but certain models have emerged as frontrunners in digital marketing. Let’s talk about the most influential marketing AI models you’re likely to encounter in your marketing efforts.
How Many AI Models Are There?
I’d love to give you a definitive answer to this question, but the truth is, AI technology is changing daily.
There are hundreds, possibly thousands, of distinct models, with new ones emerging weekly. This proliferation spans from niche, task-specific models to broad, multi-purpose systems.
In digital marketing, we see models tailored for everything from customer segmentation to content optimization. While tracking every model is impossible, understanding the main types and standout examples is crucial.
Let’s dig into a few common names you’ll see.
1. GPT-4o
Imagine an AI that can write, analyze, and problem-solve across countless domains. That’s GPT-4o, OpenAI’s latest LLM, that can generate human-like text and understand complex contexts.
From crafting compelling ad copy to producing in-depth blog posts, GPT-4o is a content creation powerhouse. It also shines in data analysis and customer interaction scenarios.
One of GPT-4o’s predecessors, GPT-3.5, initially powered ChatGPT, the chatbot that exploded onto the scene in late 2022 and brought the world’s attention to the power of LLMs and generative AI.
With ChatGPT, millions of people saw how AI can help with everyday tasks and creative processes in practical ways. This surge in popularity has accelerated the adoption of AI in marketing and opened new avenues for customer engagement and content strategy. You can use the GPT-4o model when you sign up for the paid version of ChatGPT.
2. Gemini
Gemini represents Google’s latest leap in AI technology, building on the foundation of its predecessor, Bard. While Bard was primarily a text-based AI chatbot competing with ChatGPT, Gemini goes several steps further.
This advanced AI model is designed to be multimodal, which means it seamlessly processes and generates a variety of types of content, including text, images, and code.
For marketers, Gemini opens up a world of possibilities. It inherits Bard’s strengths in information retrieval and task assistance, which are invaluable for market research and customer support.
Then its multimodal capabilities can analyze visual data alongside text, create diverse content types, and provide more comprehensive insights by integrating multiple data formats.
Marketers can use Gemini for everything from generating product descriptions with matching visuals to analyzing customer feedback across text and image-based platforms.
3. Claude
Claude, developed by Anthropic, is an AI assistant known for its strong language understanding and generation capabilities. It excels in tasks like content creation, analysis, and problem-solving.
In marketing, Claude can be used for SEO copywriting, answering complex queries, and assisting with research. Its strength lies in providing detailed, nuanced responses, making it valuable for in-depth content creation and strategy development.
Anthropic, which former OpenAI researchers founded, developed Claude using a novel AI training approach called constitutional AI. This method aims to create AI systems more aligned with human values and ethical considerations.
As a result, Claude is known for engaging in thoughtful discussions on complex topics, maintaining consistency in long conversations, and adhering to ethical guidelines. For marketers, this translates to an AI assistant that can handle sensitive issues with care and provide well-reasoned, contextually appropriate content.
4. Random Forest
Random Forest is a marketer’s Swiss Army knife when predicting customer behavior or segmenting your audience. This versatile machine-learning algorithm uses multiple decision trees to tackle complex datasets easily. It excels at handling numerous variables, making it perfect for analyzing diverse marketing data.
Leo Breiman and Adele Cutler developed Random Forest in 2001, and the algorithm has stood the test of time in the field of machine learning (ML). It builds upon the concept of decision trees, combining many trees to create a “forest” that’s more accurate and robust than any single tree. This ensemble approach helps mitigate overfitting, a common problem in predictive modeling.
One of its key strengths is providing importance rankings that help you understand which factors most influence outcomes. Random Forest is an invaluable tool in your AI arsenal for data-driven marketing strategies and resource allocation.
5. GPT-4o Image Generation
DALL·E’s legacy continues with GPT-4o, OpenAI’s newest model that takes AI-generated imagery to the next level. This multimodal model can create original, realistic images and art from text descriptions. It’s like having an AI artist on your marketing team capable of bringing your wildest visual concepts to life with just a text prompt.
The model was introduced in early 2025. It is a successor to the DALL·E 2 model, which was released in 2022. GPT-4o builds on its legacy by making image generation faster, more interactive, and easier to use in everyday marketing workflow.
For marketers, this opens up a world of possibilities in content creation and brand visualization. Need a unique image for a blog post? Want to mock up product concepts quickly? GPT-4o can handle these tasks with surprising creativity and accuracy.
How to Use AI Models
Now it’s time to learn how to use different types of AI models in our day-to-day work. In this section, I’ll touch on some practical applications of AI in digital marketing.
AI-Driven Personalization
AI models are transforming personalization from a buzzword to a reality. By analyzing vast amounts of user data, these models can predict individual preferences and behaviors with remarkable accuracy. This allows us to tailor content, product recommendations, and marketing campaigns to each user’s specific interests and needs.
For example, an e-commerce site might use AI to dynamically adjust its homepage for each visitor and showcase products they’re most likely to buy. The result? Higher engagement rates, improved customer satisfaction, and increased conversions. However, it’s crucial to balance personalization with privacy concerns. Always ensure you’re using data responsibly and transparently.
The Power of Predictive Analytics
Predictive analytics is where AI truly shines in marketing. Based on historical data, these models can forecast future trends, customer behaviors, and campaign performance. For instance, you can predict which leads are most likely to convert so you can focus your efforts where they’ll have the biggest impact.
AI can also forecast demand for products or services, which helps you optimize inventory and ad spend. In SEO, predictive models can even anticipate algorithm changes or emerging search trends, giving you a substantial competitive edge.
Precision Marketing with AI Customer Segmentation
AI-driven customer segmentation takes targeting to a new level. Instead of relying on basic demographics, AI models can identify complex patterns in customer behavior, preferences, and interactions. This allows you to create highly specific segments for more targeted marketing efforts.
For example, an AI model might identify a segment of customers who prefer eco-friendly products and are most responsive to email campaigns on Tuesdays. You can use this information to tailor your messaging and timing to this group for maximum impact. You’ll get more bang for your buck with every campaign.
The New Face of Customer Service
AI-powered chatbots and virtual assistants are significantly improving customer service and engagement. These tools can handle a wide range of customer inquiries 24/7, provide instant responses, and free up human agents for more complex issues. Advanced models can understand context and nuance to offer personalized recommendations or troubleshooting steps.
They can also gather valuable data on customer preferences and pain points. In marketing, you can use chatbots to qualify leads, guide customers through the sales funnel, or even assist with product selection. The key is to design these tools to complement, not replace, human interaction, and give customers multiple options for interacting with your customer service team (in case they’re not big fans of chatbots).
AI Can Be Your Content Creation Copilot
Models like GPT-4o can generate draft text content, from social media posts to long-form articles, potentially providing inspiration and saving time.
However, it’s important to note that AI-generated text can often sound stilted or stiff. If you’re going to use AI models to generate content, factor in ample time for editing to ensure the content has a natural narrative flow and sounds authentically human.
AI can also analyze existing content to suggest improvements for SEO, readability, or engagement. For example, it might recommend keyword optimizations or identify topics that resonate with your audience. GPT-4o and other AI models can also be powerful tools for imaging creation and editing.
Remember that human creativity and oversight remain crucial throughout the content creation process, whether you’re working with text or images. AI should be seen as a tool to enhance, not replace, human content creation skills.
The most effective approach combines AI’s efficiency and analytical capabilities with human creativity, judgment, and brand understanding.
The Challenges Ahead for Full AI Adoption
AI isn’t a magic bullet for marketing challenges. As we push towards fuller adoption, we’re encountering significant obstacles.
Let’s explore the real-world difficulties of integrating AI models into marketing strategies and operations.
The Ethical Tightrope of AI-Driven Marketing
AI models raise significant ethical concerns in marketing. Data privacy is a primary issue, as these models often require vast amounts of personal information to function effectively. There’s a fine line between personalization and intrusion.
Bias in AI models is another critical concern. Skewed training data can lead to discriminatory outcomes in targeting or content creation.
Transparency is also challenging. Many AI models operate as “black boxes,” which makes it difficult to explain decisions to customers or regulators. As marketers, you must navigate these ethical waters carefully and ensure your AI use aligns with privacy laws, fairness principles, and your brand values.
AI Integration and Implementation Is More Than Just Plug-and-Play
Integrating AI models into existing marketing infrastructures is no small feat. Many organizations struggle with legacy systems that aren’t designed to work with advanced AI. Data silos can prevent AI models from accessing the comprehensive information they need.
There’s also a significant skills gap. Many marketing teams lack the technical expertise to implement and manage AI systems. This often leads to a reliance on third-party vendors, which can create challenges around data control and customization.
Successful integration requires a strategic approach that involves cross-departmental collaboration and thoughtful resource allocation.
The Costs and ROI of AI in Marketing
The financial aspect of AI adoption in marketing is a double-edged sword. Initial investment in AI technology can be substantial and include costs for software, hardware, data preparation, and skilled personnel.
These upfront costs can be prohibitive for many businesses, especially smaller ones. Measuring ROI and doing SEO reporting when AI is involved is also complex. While AI can lead to efficiencies and insights, quantifying its exact impact on marketing outcomes may be challenging.
Some AI benefits, like improved customer experience, may not translate to immediate increases in conversions. You’ll need to develop robust frameworks for assessing AI’s long-term value and short-term costs against potential future gains.
AI’s Achilles’ Heel: Technical Limitations
Despite rapid advancements, AI models still face significant technical limitations. Many models struggle with understanding context and nuance, leading to errors in content generation or customer interactions.
Scalability can also be an issue, especially for real-time applications like personalized web experiences. AI models often require substantial computing power, which can be a bottleneck for complex tasks.
Data quality remains a critical challenge. Models are only as good as the data they’re trained on, and obtaining clean, relevant data can be difficult. Additionally, the “black box” nature of some advanced models makes troubleshooting and fine-tuning challenging.
Learn More About AI Models in TTT Academy
The world of AI in digital marketing is changing at breakneck speed, and you’ll need to stay ahead of the curve if you want to leverage these powerful tools effectively. That’s where TTT Academy comes in.
Our courses cover everything from AI basics to advanced applications, and we’re constantly updating our materials to reflect the latest trends.
Here are a few of our AI lessons in TTT Academy:
- 7 Ways Ian Howells Is Using AI in Workflows
- Competitive Analysis Using AI
- How to Use AI for SEO Edits
But we don’t stop at courses. With the Full Accelerator membership, you get access to our exclusive TTT Slack Channel. This is where the real magic happens. On Slack, you can engage in ongoing discussions about the latest AI developments, share experiences, and learn from other SEO and marketing experts.
Learn more about TTT Academy here.
Semrush
- Semrush – Best overall SEO functionality
- Google Search Console – Best free (partial) data from Google
- Advanced Web Ranking – Best for reporting
- SERPWatcher by Mangools – Best for bloggers and small teams
- Ahrefs – Best for keyword analysis
- SEO PowerSuite – Best affordable option
- SEO Monitor – Best for forecasting
- Local Viking – Best for local SEO map tracking
- Nozzle – Best for data visualization
- ProRankTracker – Best for agencies and SEO professionals